Machine Learning Algorithms and Their Impact on Digital Wallet Security
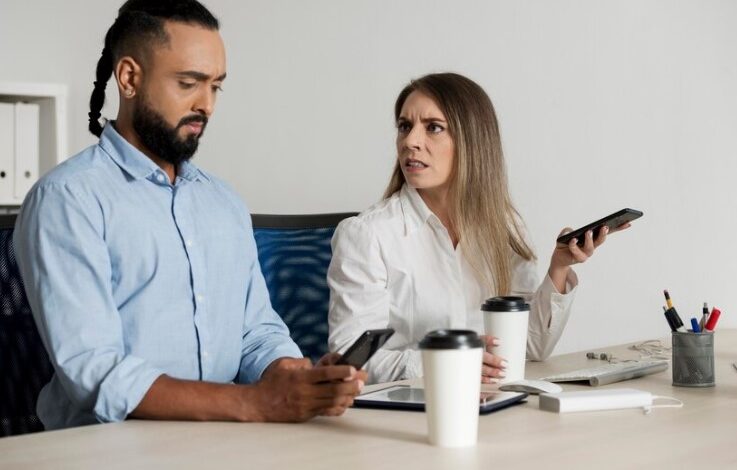
In the rapidly evolving world of digital finance, security remains a top concern for users and providers alike. Digital wallets have become a critical tool for managing transactions, investments, and personal finances. As cyber threats grow more sophisticated, enhancing the security of digital wallets is paramount. Machine learning (ML) algorithms are playing a crucial role in this endeavor, significantly improving the security protocols of digital wallets. This blog post explores how machine learning algorithms impact digital wallet security and their relevance to bitfunded users.
Advanced Fraud Detection
One of the most significant contributions of machine learning algorithms to digital wallet security is advanced fraud detection. Traditional security measures, such as rule-based systems, often struggle to keep up with evolving fraud tactics. Machine learning algorithms, however, excel at identifying patterns and anomalies in large datasets.
ML models can analyze transaction data in real-time, learning from historical patterns to detect unusual behavior. For bitfunded users, this means enhanced protection against fraudulent transactions. Machine learning algorithms can identify suspicious activities, such as unauthorized access or unusual transaction amounts, and trigger alerts or preventive actions to safeguard user accounts.
Real-Time Threat Detection
Machine learning algorithms enable real-time threat detection by continuously monitoring and analyzing digital wallet activities. Unlike traditional security systems that may rely on periodic scans or updates, ML algorithms provide dynamic and ongoing protection.
For bitfunded users, real-time threat detection is crucial in managing and securing financial transactions. ML algorithms can instantly flag potential security threats, such as attempts to access accounts from unfamiliar devices or locations. This rapid response capability ensures that potential security breaches are addressed promptly, minimizing the risk of financial loss.
Behavioral Analysis
Behavioral analysis is another area where machine learning algorithms have a profound impact. ML models can analyze user behavior, such as spending patterns, transaction frequency, and login habits, to build a profile of typical user behavior. This profile helps in detecting deviations that may indicate fraudulent activities.
For bitfunded users, behavioral analysis enhances security by identifying anomalies that deviate from normal trading or transaction patterns. If an unusual pattern is detected—such as a sudden surge in transaction volume or access from an atypical location—the system can prompt additional verification steps or alert the user, preventing potential security issues.
Read also: Can I Find Information on Cryptocurrency Investments on How2Invest?
Adaptive Security Measures
Machine learning algorithms enable adaptive security measures, allowing digital wallets to continuously evolve and improve their security protocols. ML models can update their algorithms based on new data and emerging threats, ensuring that security measures remain effective in a constantly changing threat landscape.
For bitfunded users, adaptive security means that their digital wallets are equipped to handle the latest security challenges. As new fraud techniques and cyber threats emerge, machine learning algorithms can adjust and enhance security measures, providing ongoing protection against evolving risks.
Risk Assessment and Management
Machine learning algorithms also contribute to risk assessment and management by analyzing various factors that influence security. ML models can evaluate risk based on transaction history, user behavior, and external threat data, providing insights into potential vulnerabilities.
For bitfunded users, risk assessment helps in identifying and mitigating potential security threats before they escalate. By evaluating risk factors and providing recommendations for risk management, machine learning algorithms enable users to make informed decisions about securing their digital wallets and financial assets.
Enhanced User Authentication
ML algorithms enhance user authentication by incorporating advanced techniques such as biometric recognition and multi-factor authentication. Machine learning models can analyze biometric data, such as fingerprints or facial recognition, to verify user identities with high accuracy.
For bitfunded users, enhanced authentication methods provide an additional layer of security, ensuring that only authorized individuals can access their digital wallets. By combining biometric recognition with other authentication methods, such as OTPs or device-based verification, machine learning algorithms help protect against unauthorized access and potential security breaches.
Future Prospects
As machine learning technology continues to advance, its impact on digital wallet security will likely grow. Future developments may include even more sophisticated algorithms for detecting and mitigating threats, as well as integration with emerging technologies such as quantum computing and advanced encryption methods.
For bitfunded users, the future of machine learning in digital wallet security promises enhanced protection, more accurate threat detection, and improved risk management. As machine learning algorithms evolve, they will continue to play a pivotal role in safeguarding digital wallets and ensuring the security of financial transactions.
Conclusion
Machine learning algorithms are revolutionizing digital wallet security by providing advanced fraud detection, real-time threat monitoring, behavioral analysis, and adaptive security measures. For bitfunded users, these advancements offer significant benefits in protecting financial assets and managing risks. As technology continues to evolve, machine learning will remain a critical component in enhancing digital wallet security and safeguarding against emerging threats. By leveraging the power of machine learning, digital wallets can provide robust protection and a secure financial experience for users worldwide.